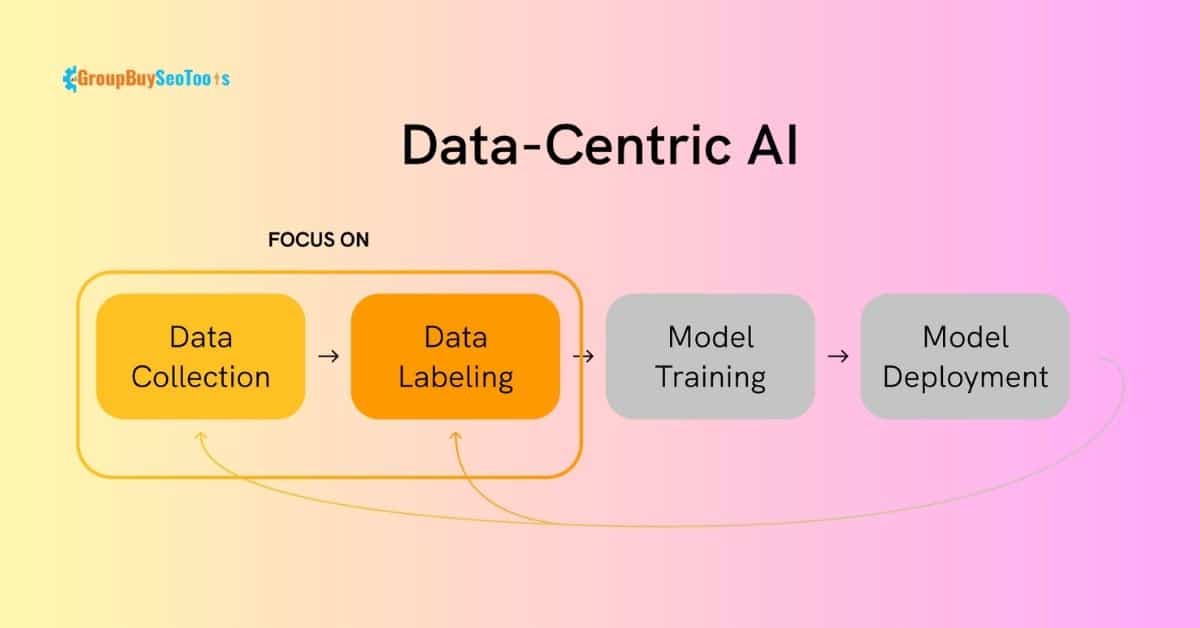
Data Centric ai
Data-centric AI (DCAI), a cutting-edge branch of AI era, is concerned with expertise, the use of, and drawing conclusions from facts. AI changed into once heavily depending on hints and heuristics in advance than becoming information-centric. These is probably beneficial in some occasions, but even as used on fresh data sets, they frequently produce much less-than-perfect outcomes or errors.
By adding tool analyzing and massive facts analytics equipment, statistics-centric Artificial Intelligence modifies this through allowing it to investigate from statistics rather than relying on algorithms. It can for that reason make wiser options and deliver more particular effects. It moreover has the functionality to scale up a long way more than conventional AI techniques do. Data-centric AI will possibly keep growing in importance as statistics units get larger and more complicated.
What is Data Centric Architecture in AI?
The facts-centric approach includes meticulously optimising datasets to elevate the accuracy of AI structures. This strategy has capability, in step with machine analyzing professionals, due to the truth processed information produces better results than uncooked facts. A information-centric method locations awesome statistics input beforehand of converting the model’s parameters.
In gadget mastering, tagged photographs, textual content, audio documents, movies, and one of a kind information are used as training records. If the schooling information is awful, the created model and its optimisation will perform poorly. With AI-powered chatbots, this could bring about terrible consumer reports, but it may be disastrous for natural algorithms or unbiased automobiles.
How Does Data Centric AI Work?
Data augmentation, interpolation, and extrapolation are 3 strategies utilized by facts-centric AI to comply in your corporation’s necessities.
Using facts-centric AI, you do not need to educate a model on a particular dataset. Instead, the device makes a easy prediction from the education data provided thru your enterprise. This manner that a model evolved using facts out of your business agency will probable moreover artwork well with one-of-a-kind datasets.
You may additionally additionally decorate the awesome of your fashions through manner of developing extra instances of an modern-day instance thru extrapolation or interpolation. Additionally, it entails growing new facts instances from older ones. Either extrapolation or interpolation may be used to finish this mission.
The following steps make up a records-centric AI technique in famous:
- Labelling your datasets efficiently and solving any errors
- casting off noisy facts times from the analysis
- Feature Engineering Error Analysis for Data Augmentation
- Better consequences can be acquired with the resource of using area professionals to evaluate the accuracy or consistency of data factors.
Data Centric AI Architecture Advantages
- Enhance Performance. A information-centric approach includes growing AI systems with exquisite statistics, ensuring that the records transmits the statistics the AI wants to learn. In addition to reducing wasteful trial and mistakes time spent growing the version without enhancing inconsistent data in a particular facts set, this aids teams in accomplishing the needed overall performance level.
- When first-rate control is statistics-centric, collaboration is superior amongst managers, professionals, and developers. They can collaborate whilst growing defects or tags with the intention to be regular via agreeing on them or with the resource of developing a model earlier than reading the consequences if you want to perform extra optimisations.
- Data-centric AI accelerates improvement considering agencies may go concurrently and right now impact the facts that the AI device makes use of.
Data Centric AI Architecture Disadvantages
- It is probably tough to reveal and manipulate the great of statistics.
- If statistics units don’t correctly replicate the populace, they may be prejudiced.
- This method can be steeply-priced because a number of facts is wanted to educate the models.
Conclusion
The results of fashions are extra accurate way to facts-centric AI, which additionally opens up new applications for this concept. As developers running with AI place a extra emphasis on models than information, it is gaining traction. The first-rate of the input records is now more regularly considered while enhancing effects than inside the beyond when engineers hired model-centric strategies to increase the outcomes and accuracy of version predictions.